Perception is in the Eye of the Beholder
(Posted on Thursday, July 13, 2023)
Originally published on Psychology Today on 7/6/2023
How optical illusions work has been long-debated among scientists and philosophers who wonder whether these illusions stem from neural processing in the eye or involve higher-level cognitive processes such as context and prior knowledge.
New research has found that many visual illusions are caused by limits in how our eyes and visual neurons work instead of more complex psychological processes. Scientists examined illusions in which an object’s surroundings affect how we see its color or pattern to evaluate whether these illusions are caused by neural processing in the eye and visual centers in the brain or involve higher-level mental processes such as context and prior knowledge.
A Quick Look at the Eye
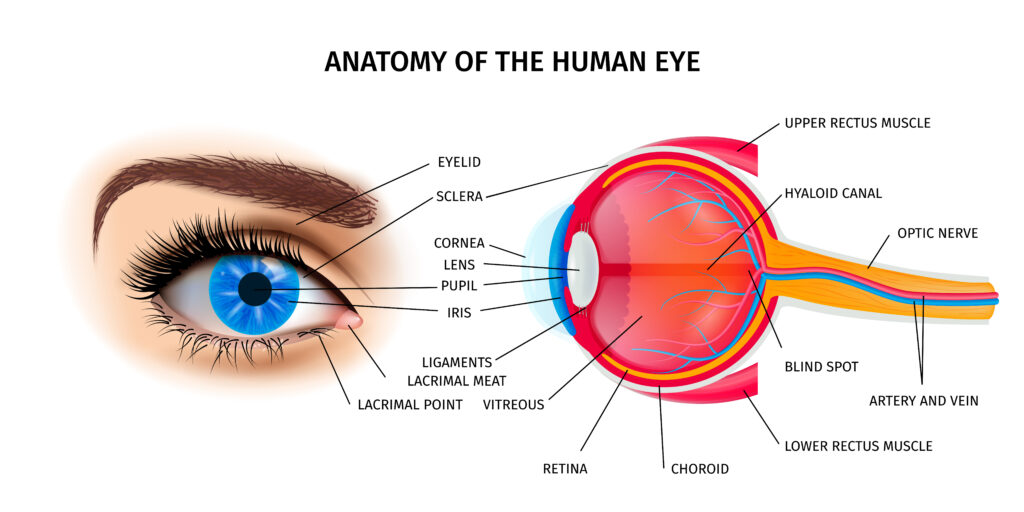
Human eye anatomy poster with eyelid and optic nerve symbols isometric vector illustration
The human eye is an incredible feat of biology, intricately designed to absorb and transmit light through a series of components to the brain for interpretation. The cornea and lens work cohesively to bend and focus the incoming light entering the pupil. The iris, or the colorful part of the eye, regulates the amount of light let into the eye by controlling the size of the pupil.
The light focuses directly onto the retina, a group of cells at the back of the eye responsible for transmitting signals to the brain. The retina contains two types of light-sensitive cells: rods and cones. Rods are highly sensitive and are responsible for detecting dim light, while cones respond best to bright light and are involved in color vision.
As light enters the eye, it is absorbed by the rods and cones in the retina, where it is transformed into electrical signals that travel along the optic nerve to the brain. The brain then processes the signals into images we can see and interpret.
In the Mind’s Eye: Neural Processing
While our eyes can take in an incredible amount of information, the brain can only process so much at once, leading to shortcuts that simplify what we see. This can result in situations where we perceive things that aren’t there, such as optical illusions.
The brain relies on previous experiences and knowledge to make assumptions about what we see, leading to perceptions that may not match reality. Or, that’s what we thought was happening when we saw optical illusions, but new research suggests it may be more straightforward.
Researchers from the University of Exeter developed a model that suggests limits to neural responses, not deeper psychological processes, explain how we process optical illusions. The model combines “limited bandwidth” with information on how humans perceive patterns at different scales. It was developed to predict how animals see color, but it can predict many visual illusions seen by humans correctly.
The team developed a computational model based on efficient coding, and it considers the limited neural bandwidth and contrast sensitivity functions to predict color appearance. This simple and generalizable model could predict almost all visual phenomena and illusions without requiring high-level processes. This suggests that limited bandwidth and efficient coding can explain many complex visual phenomena.
A Model for Simulating Vision
A comprehensive understanding of color appearance is crucial for accurate modeling and application in various fields. The visual system can efficiently encode images of natural scenes due to photoreceptor adaptation and lateral inhibition. This allows for the optimal use of limited neural bandwidth and metabolic energy, a fundamental principle in early visual processing.
A Spatiochromatic Bandwidth Limited, or SBL, model of early vision has been developed, which uses luminance, the sense of light and darkness, and chromatic spatial filters to cover the detectable range of spatial frequencies, how the retina determines something’s relative location.
This model predicts color and lightness and offers a framework for understanding neural image processing. The SBL model is designed to simulate the human visual system’s information processing. It achieves this through a series of steps.
In the first step, the image is filtered with spatial filters. Then the signal power in each channel is normalized to the filter’s response to a natural scene. The last step is where the filter outputs are summed to recover their representation of the original image.
The model comprises three spectral classes of filter that correspond to those in human vision: achromatic/luminance, blue-yellow, and red-green. For the latter two, the model assumes that the filters are less orientation selective.
With these steps and spectral classes, the SBL model comprehensively simulates visual information processing, incorporating multiple filters and thresholds. Its implementation includes parameters based on psychophysical measurements.
Applying the Model and Finding Answers
The study by researchers at the University of Exeter tested the SBL model for its ability to account for 52 perceptual phenomena that visual mechanisms could explain. It correctly predicted the direction of almost all effects with no free parameters and can be adjusted to predict all effects.
The SBL model is a feed-forward model, meaning it does not require feedback loops for normalization and can explain many visual phenomena without delay. The model does not invoke light adaptation or eye movements and implies that color constancy is mainly independent of the adaptation state of the photoreceptors.
The study yields invaluable insights into the performance of the SBL model. The findings suggest that grasping the concepts of limited bandwidth and efficient coding may unlock the secrets behind complex visual phenomena. By investigating visual perception, we inch closer to unraveling the mystery of how the human eye perceives and comprehends the world around us.
The study highlights the crucial role of neural responses in visual illusions. Given its feed-forward architecture devoid of feedback loops, the model’s high accuracy in predicting visual effects demonstrates its significance in understanding perception. For progress in artificial intelligence and perception-wise technologies, scaling up the limitations of neural responses in natural scenes is vital. This study lays a solid foundation for progress in visual perception, providing deeper insights into the mechanisms that control optical illusions.